XPENG Launches 72B-Parameter Self-Driving Model, Validates Scaling Law for Autonomy
- Max
- Apr 14
- 3 min read
On April 14, at an AI technology sharing event in Hong Kong, XPeng Motors announced a major R&D initiative: the development of a 72-billion-parameter autonomous driving foundation model, dubbed the 'World Base Model'. This large-scale model will serve as the core for Level 4 autonomous driving and, through a distillation-based deployment method, will be implemented across vehicle platforms—eventually becoming the unified AI brain for XPeng's vehicles, robots, and flying devices.

“The 'World Base Model' is the cornerstone for XPeng’s journey toward L3 and L4 autonomous driving,” said Li Liyun, Head of Autonomous Driving at XPeng. “It won’t just power our cars—it will be a general-purpose model for all of our physical AI terminals in the future.”
Built on a large language model architecture, 'World Base Model' is trained with multimodal data including vision, navigation, and driving behavior. It possesses the capabilities for scene understanding, decision-making, and action generation. Critically, it features chain-of-thought (CoT) reasoning, enabling it to interpret complex scenarios and translate them into driving actions such as steering and braking—much like a human would.
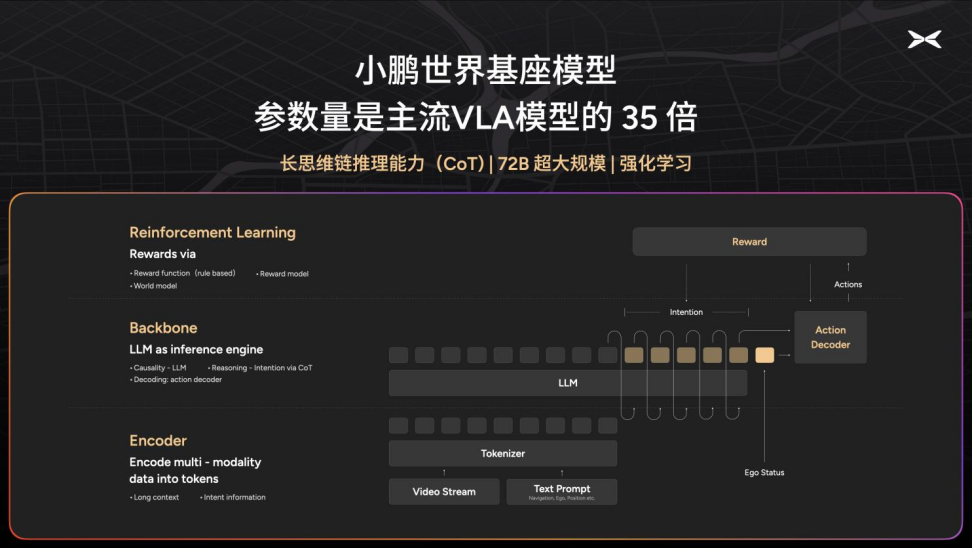
Building a "Cloud-Based Model Factory" from Scratch: Iterations Every Five Days
To support the training and deployment of this model, XPeng began building its AI infrastructure in 2024. The company has constructed the first 10-EFLOPS compute cluster in China's automotive industry, with over 10,000 GPUs and a sustained utilization rate exceeding 90%.
This is more than just “stacking hardware.” XPeng has established a full-scale cloud-based model factory, encompassing pre-training, post-training, reinforcement learning, distillation, and deployment. With this setup, the model iteration cycle has been compressed to just five days.
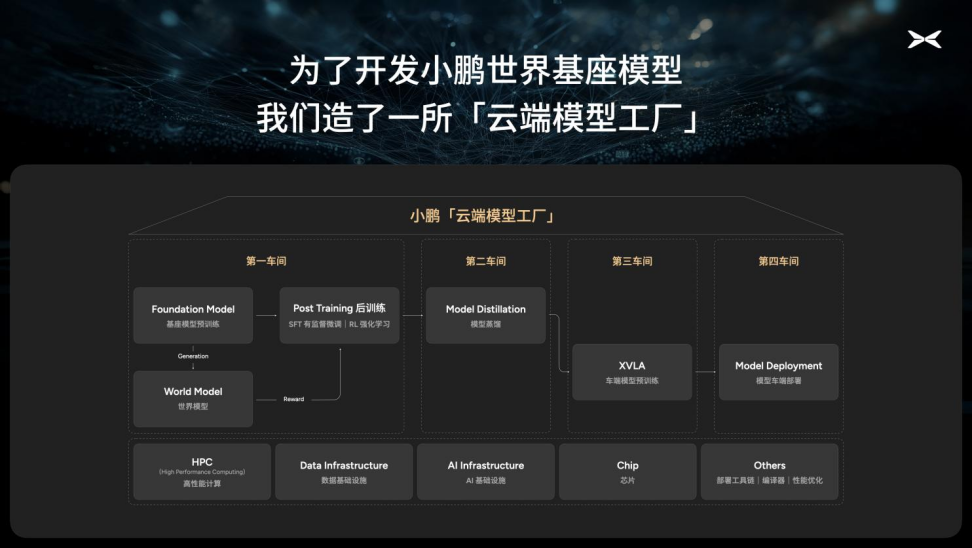
In tandem, XPeng developed its own data infrastructure platform, improving video data upload efficiency by 22x, training bandwidth by 15x, and overall model training speed by 5x. The company has already amassed over 20 million driving clips and is targeting 200 million by the end of the year.
“You can’t solve training challenges just by buying GPUs,” said Dr. Liu, the head of the ‘World Base Model’ project. “We invested a lot of effort into optimizing data flow—that’s the key to efficient model training.”
Three Key Milestones: From Theory to On-Vehicle Testing
Over the past year, XPeng has achieved three critical milestones in foundation model development:
Scaling Law Proven Effective in Autonomous Driving

While scaling laws—where model performance improves with more parameters and data—have been validated in NLP, autonomous driving is far more complex due to its multimodal nature.
“We tested models with 1B, 3B, 7B, and up to 72B parameters,” said Dr. Liu. “The scaling laws still apply: larger models indeed perform better.”
Successful Implementation of Base Model Control in Vehicles with Aftermarket Compute Units
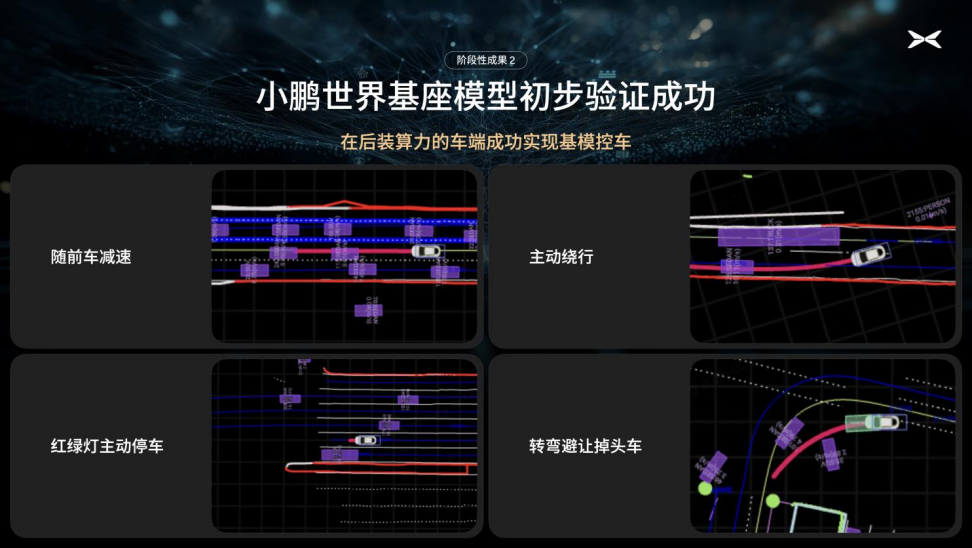
In early tests, XPeng has successfully deployed smaller versions of the foundation model on real vehicles equipped with aftermarket compute units. Even with limited model size, the system could execute basic driving tasks—proving the feasibility of deploying large-scale cloud-trained models on vehicles.
Launched 72B Parameter Training and Reinforcement Learning Framework
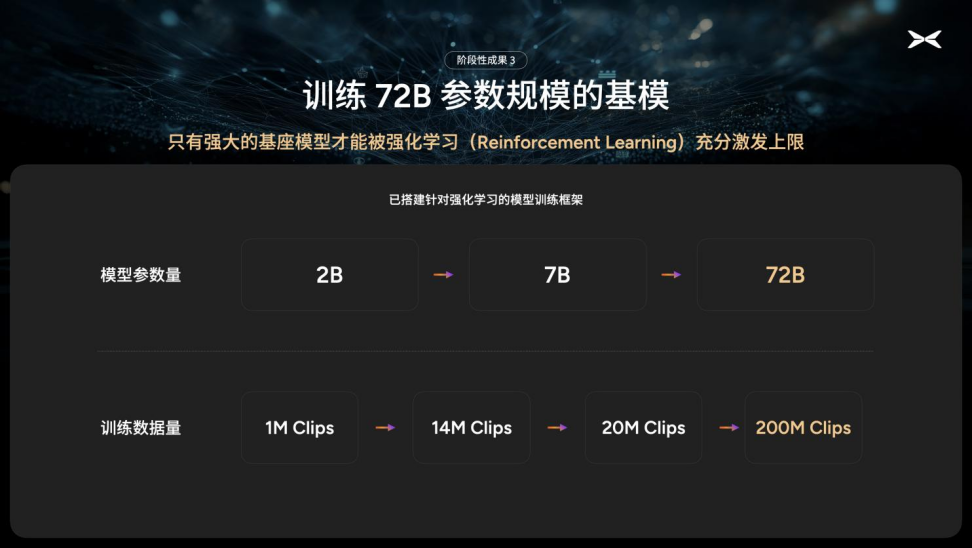
XPeng has also begun building a reinforcement learning framework to address long-tail scenarios—rare and extreme traffic conditions not well represented in training data.
“Reinforcement learning allows the model to evolve and handle unfamiliar situations,” said Dr. Liu.
The reward model design draws from XPeng’s earlier rule-based systems, transforming manually encoded driving rules into incentives for reinforcement learning. This helps the model learn safe driving behavior faster.
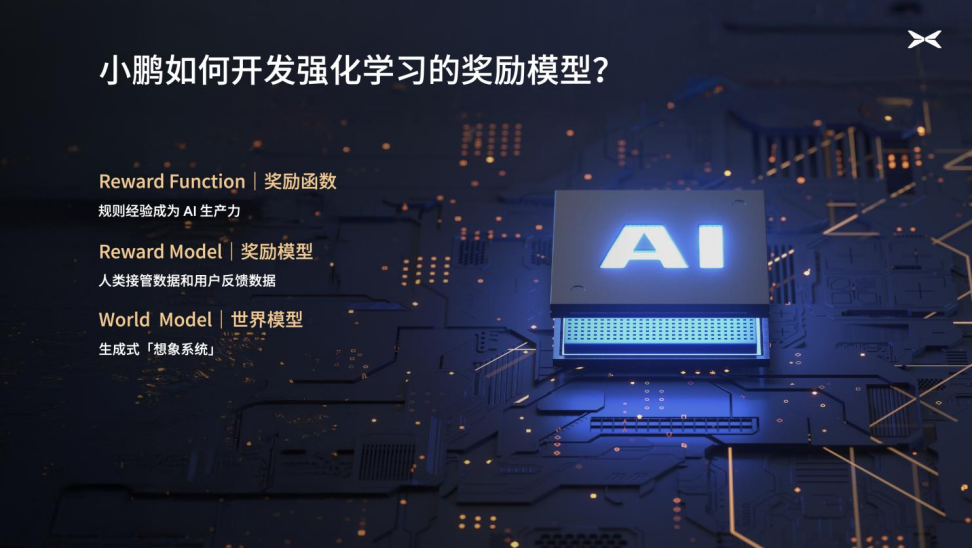
In parallel, XPeng is developing a “world model” system—a virtual environment that simulates the cause-and-effect feedback of real-world driving. This system enables the foundation model to rehearse and adapt to complex scenarios in advance, accelerating its evolution.
XPeng plans to share further technical details about these advancements at the CVPR international conference in June 2025.
Comments